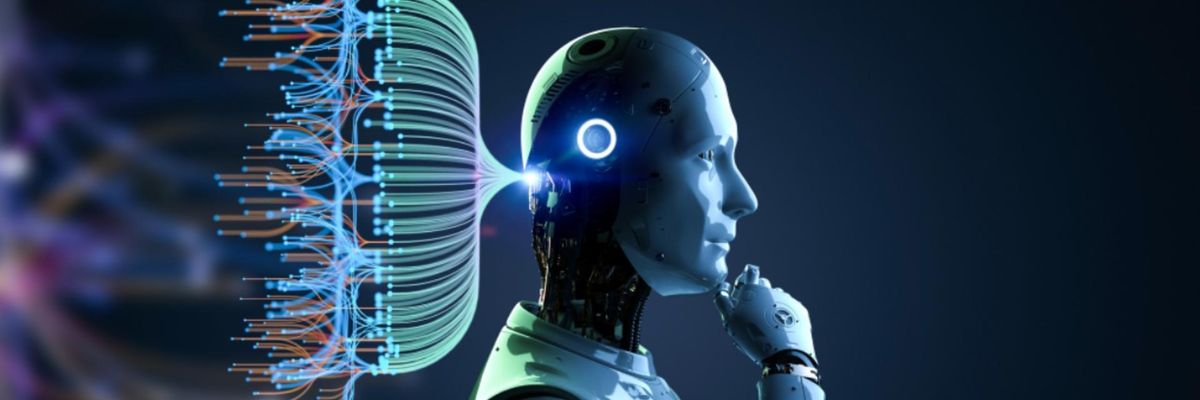
AI adoption in business: what are the challenges and how to overcome them?
Ricoh Latin America presentamos cinco barreras de adopción de IA y cómo las empresas pueden superarlas de cara al 2020.
Julio Urrutia, Director Consulting at Ricoh Latin America
Manual data entry, spam detection, inaccurate medical diagnostics, poor customer segmentation, and predictive maintenance are some of the business problems that many companies experience daily, but as more organizations adopt Artificial Intelligence in their multiple business processes, the visibility of the business benefits of this disruptive technology increases and helps facilitate better decision making. According to a recent study1, global AI industry revenues are expected to grow from $8.1 billion in 2018 to $105.8 billion by 2025.
At Ricoh, we're working to realize its full potential. This year, we conducted a proof-of-concept implementation of AI in our manufacturing processes which saved the company an estimated $400,000.00 per year in manufacturing costs. But surely, as the saying goes, "a journey of a thousand miles begins with a single step". Translating this phrase to the business world, these first steps can often be the most challenging.
From legacy systems to securing investment and even implementing AI in the workplace, several challenges need to be overcome early in the process. Against this backdrop, Ricoh Latin America presents five barriers to AI adoption and how businesses can overcome them in the run-up to 2020:
1) Legacy systems: Many organizations rely on legacy infrastructure, applications, or devices to deliver some of their IT operations. Upgrading all at once is a major challenge. This legacy infrastructure is often seen as an impediment to adopting machine learning or artificial intelligence. Fortunately, cloud computing, or more specifically, hybrid cloud, has changed this situation. A hybrid cloud is when an organization uses both on-premises and cloud-hosted IT infrastructure. This is an increasingly common situation.
Embracing artificial intelligence and machine learning does not mean that the entire IT area needs to be upgraded. But it does require embracing the cloud for data analytics and artificial intelligence. Modern data lake technology works well in a hybrid environment, where the cloud can be used for analytics with on-premises operating systems. The other advantage of cloud solutions is that updates and new features are deployed automatically, leading to fewer misconfigurations, security vulnerabilities, and incompatibilities, which is important to consider when implementing emerging technologies.
2) Skills shortage: There is currently a skills shortage around AI and machine learning, and this can hamper efforts to develop its full capabilities. It is important to give people within the business (and not just IT!) the opportunity to get involved. This is a great way to find people who are excited about AI and want to learn more.
You also need to create the right environment to develop and nurture an innovative culture. From driving collaboration to making sure people have time to explore and experiment with innovative ideas.
3) Lack of senior management support: One of the key factors to keep in mind when implementing AI to key stakeholders is that you must consider your audience and their priorities. Typically, decision-makers are not interested in the technical and operational details. They are focused on the broader business objectives and how quickly they can achieve them. Specific and realistic use cases along with projected ROI are much more useful than a "big picture". The more data and metrics you can use to support your case, the better.
4) Develop a coordinated data strategy: What I've seen working with clients is that data science projects can end up siloed. Keeping projects up and running becomes a difficult task and they are less likely to get beyond the pilot stage. The important thing is to demonstrate a robust and scalable approach.
Once the platform is ready, new models can be implemented and new areas of operation can be integrated faster, speeding up the process of making AI pervasive across the organization.
5) Lack of understanding in the business: The AI or machine learning team must be visible in the organization. Regular presentations and workshops to explain the strategy and objectives are key. This role is about explaining how it works, what it can do, and doing everything possible to include people in this journey.
There is some concern about the effects of AI on the labor market. However recent reports suggest that AI will create thousands of jobs. Demonstrating to colleagues that AI can help them, not replace them, is good practice. The dream of a 4-day work week with equal pay is a good example to share. That's something everyone would like, and AI can help us get there.